In today’s manufacturing and distribution landscape, the supply chain isn’t just a back-office function—it’s a mission-critical revenue driver. Yet many organizations still treat it as a cost center. What they don’t realize is that beneath the surface, inefficiencies, missed forecasts, and outdated systems are quietly draining millions from the bottom line.
AI in supply chain management is rewriting that narrative. By replacing guesswork with real-time intelligence, AI solutions for supply chain transformation allow companies to plug profit leaks, optimize decision-making, and turn their supply chains into a true competitive advantage. Here’s how.
Identifying Hidden Costs in Your Supply Chain
Supply chains are complex ecosystems. Without real-time insights and agile processes, cost drivers often go unnoticed. Common hidden costs include:
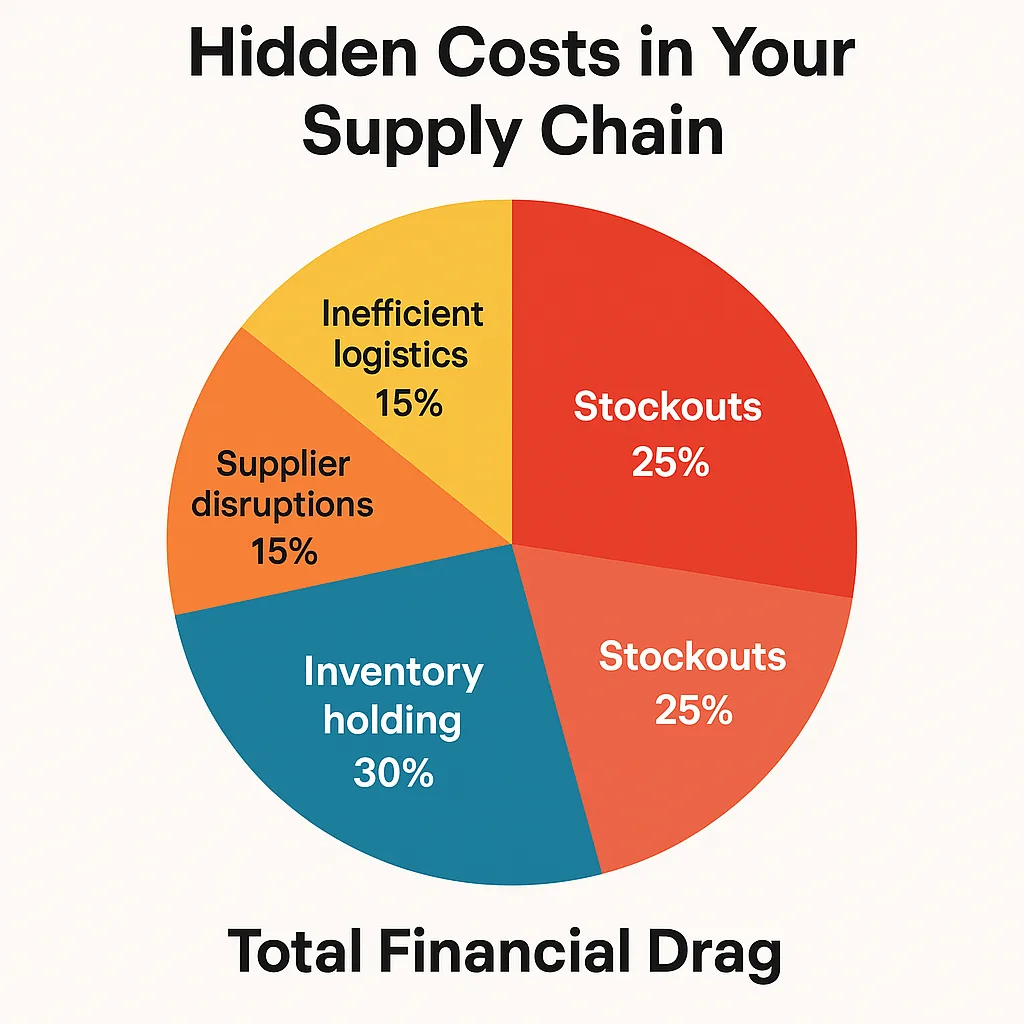
- Inventory holding and obsolescence: Excess stock ties up capital and storage resources.
- Stockouts: Lost sales and customer churn from understocking.
- Inaccurate forecasts: Lead to either wasted production or emergency replenishment.
- Inefficient logistics: Route delays, high fuel costs, and service penalties.
- Supplier performance issues: Late deliveries, quality problems, and overreliance on risky vendors.
- Data overload: Valuable time is lost manually analyzing fragmented data across siloed systems.
Each of these issues compounds into substantial financial drag—costs that can be significantly reduced using AI in supply chain operations.
Why Traditional Approaches of Supply Chain Fail to Fix These Problems
Legacy supply chain models rely heavily on manual analysis, spreadsheets, and reactive workflows. Challenges include:
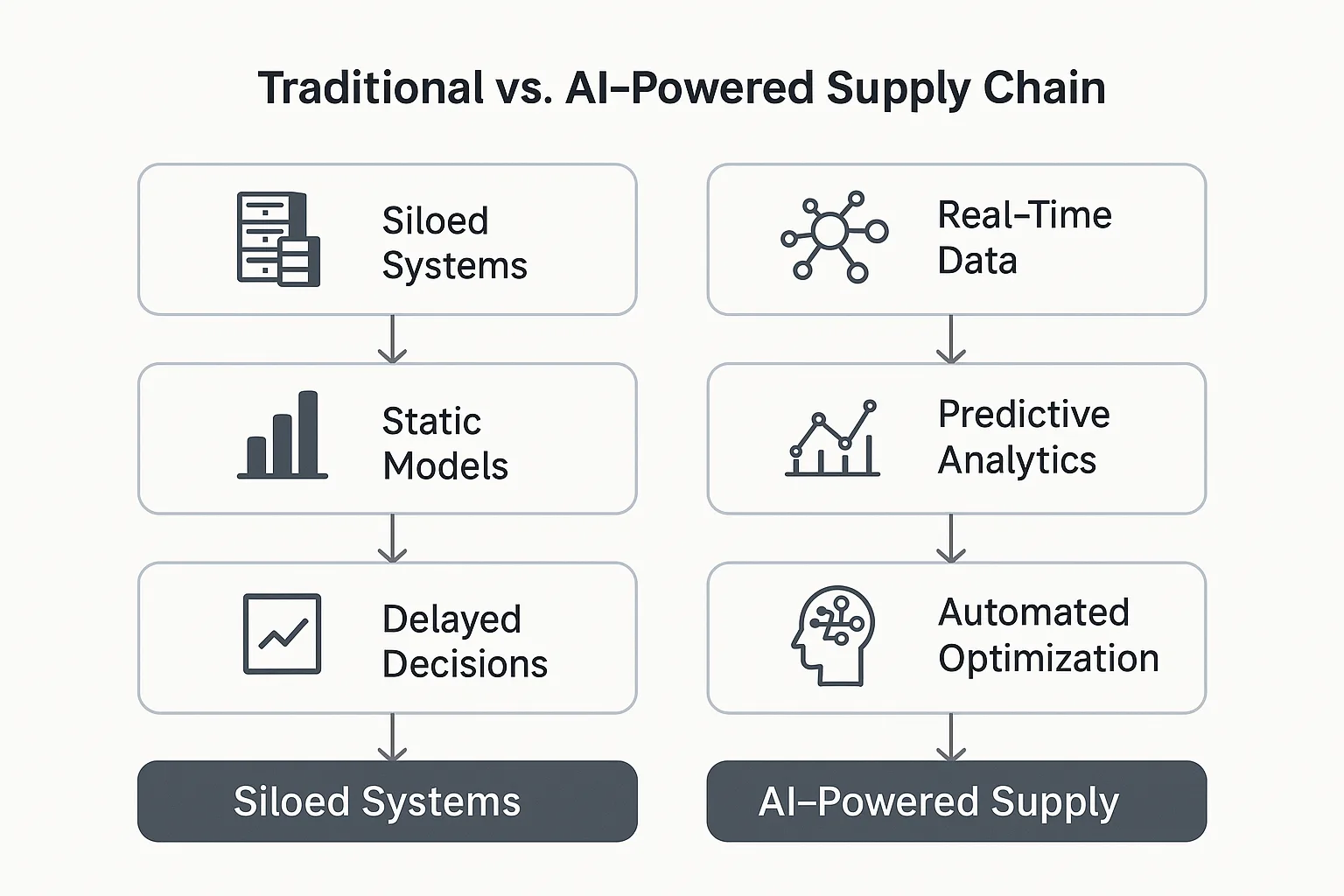
- Siloed data systems: Disconnected ERPs, WMSs, and vendor portals
- Static models: Can’t adapt quickly to market dynamics
- Lack of predictive power: Forecasts are backward-looking, not anticipatory
In a volatile, interconnected world, real-time agility and predictive analytics for logistics and procurement are no longer optional—they’re mandatory.
A New Era of AI in Supply Chain Intelligence
AI and supply chain integration transforms operations by enabling:
- Real-time data aggregation: From sales, suppliers, transportation, and operations
- Predictive analytics: For demand, delivery times, supplier reliability, and maintenance
- Automated optimization: For inventory levels, shipping routes, and replenishment schedules
- Continuous learning: Algorithms improve over time for higher accuracy
With AI for supply chain transformation, organizations become predictive, proactive, and self-correcting—replacing uncertainty with strategic foresight.
High-Impact Use Cases of AI in Supply Chain That Deliver Cost Savings
These AI applications create measurable value:
- Demand Forecasting: Anticipates customer needs using historical and external data. One of the key benefits of AI in supply chain forecasting is improved accuracy.
- Inventory Optimization: Uses dynamic inventory optimization AI to recommend ideal stock levels.
- Predictive Logistics: Models optimize routes to lower fuel use and delivery time.
- Supplier Risk Management: AI monitors and flags potential vendor disruptions.
- Automated Quality Control: Vision systems detect product defects in real-time.
These solutions enable smarter decisions and faster execution.
Real-World ROI: What Business Leaders Are Seeing
Companies using AI in the supply chain are seeing real, quantifiable returns:
- On-time delivery rates: Improve from 85% to over 95%
- Procurement savings: Average $3M+ annually in mid-sized manufacturers
- Inventory holding costs: Drop by 20–30% with better forecasting
- Stockout rates: Reduced by up to 80%, improving customer satisfaction
The Executive’s Case for AI Supply Chain Integration
AI supply chain solutions are no longer technical luxuries—they’re strategic necessities. Business leaders see:
- CFOs gaining better control over costs
- COOs achieving agility and automation
- CSCOs enjoying end-to-end visibility
- CEOs building a future-ready, tech-enabled operation
The conversation around supply chains has shifted to board-level strategy—with AI leading the charge.
How to Begin: AI in Supply Chain Management Implementation Roadmap
To adopt AI in supply chain management, start with a practical plan:
- Audit systems: Evaluate data and tech maturity.
- Prioritize use cases: Focus on pain points with the highest cost.
- Choose scalable tools: Integrate with ERP and logistics platforms.
- Pilot for proof: Test one or two high-impact applications.
- Train your teams: Make sure users can interpret and act on AI insights.
Avoiding Common Pitfalls
Even the best AI supply chain initiative can fail without the right foundation. Watch for:
- Data silos
- Poor integration planning
- Lack of stakeholder buy-in
- Short-sighted metrics
Choose experienced partners to guide you through.
Empower with Beam Data’s Approach to Intelligent Supply Chains
Beam Data delivers AI-powered supply chain automation using AI and IoT, designed for real-world impact:
- Custom AI models tailored to your KPIs
- Live dashboards for fast, clear decision-making
- ERP compatibility with SAP, Oracle, Microsoft Dynamics, and more
- Predictive insights that boost agility and reduce costs
We help enterprises build intelligent, automated supply chains that evolve with market conditions.
Your AI supply chain journey doesn’t have to be complex—but ignoring it is costly. Without intelligent forecasting, dynamic inventory optimization, and predictive analytics for logistics and procurement, your business risks lagging behind.
With AI for supply chain transformation, you can cut costs, reduce risks, and thrive through disruption. Let Beam Data help you unlock next-generation efficiency.
FAQs
What’s the typical ROI timeline for AI in supply chain?
Most companies see measurable ROI within 6 to 12 months.
Can AI work with legacy ERP or warehouse systems?
Yes—Beam Data integrates seamlessly using APIs and middleware.
How does AI improve both efficiency and sustainability?
By reducing waste, overproduction, and fuel usage, AI supports ESG and cost goals.
What’s required to get started?
Clean data, executive buy-in, and a clear use case.
How does Beam Data ensure measurable results?
Every AI project includes performance dashboards aligned to your specific KPIs.