Downtime is often seen as an unfortunate but manageable part of manufacturing. However, for business leaders, downtime is not just about idle machines—it’s about lost revenue, eroded customer trust, and delayed growth. In today’s hyper-competitive environment, even minor equipment failures can have a cascading effect on production, supply chain reliability, and profitability.
To tackle this, manufacturers are turning to AI for predictive maintenance—a smart, data-driven strategy that enables real-time equipment monitoring. This AI-based predictive maintenance approach forecasts potential failures and schedules maintenance with precision. The result: minimized unplanned downtime and a significant boost in operational efficiency.
Why is Predictive Maintenance important?
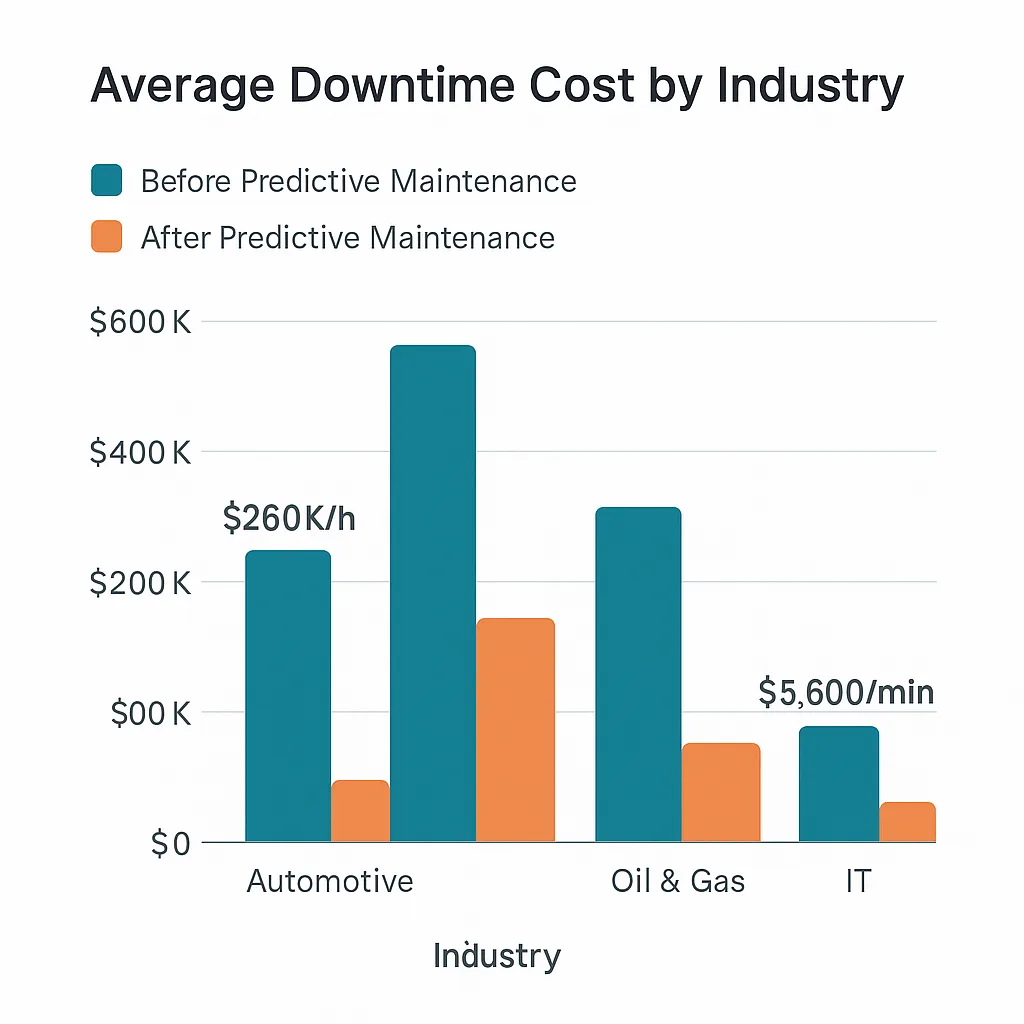
Downtime costs vary by industry and operation, but the numbers are always significant. Consider the following:
- $260,000/hour: The average cost of unplanned downtime in automotive manufacturing
- $5,600/minute: Estimated by Gartner as the average cost of IT system failure
- Up to 20% loss in annual production capacity for plants with outdated maintenance strategies
Beyond the loss of output, downtime has far-reaching effects on overall business performance. It leads to decreased customer satisfaction due to delayed deliveries, drives up operational expenses through wasted labor and overtime costs, and strains critical supply chain relationships—impacting both short-term efficiency and long-term reliability.
Every hour of unplanned downtime chips away at profitability. That’s why predictive analytics in manufacturing is more critical than ever—it turns hidden costs into clear action items.
Why Traditional Maintenance Strategies Fail to Prevent Downtime
Legacy approaches like scheduled and preventive maintenance rely on fixed timelines or technician intuition rather than actual asset conditions. This often results in three outcomes as follows. Traditional maintenance approaches often lead to inefficiencies that increase risk and cost. Over-maintenance results in replacing parts that are still functional, wasting time and resources. Under-maintenance, on the other hand, means missing subtle signs of failure until it’s too late—leading to unexpected breakdowns. These inconsistent outcomes are often driven by tribal knowledge rather than data, making maintenance reactive instead of proactive.
While reactive maintenance addresses problems after they occur, it doesn’t prevent them. A smart maintenance strategy that leverages AI and predictive maintenance transforms this reactive model into a proactive one.
What Is AI Predictive Maintenance—And How Does It Work?
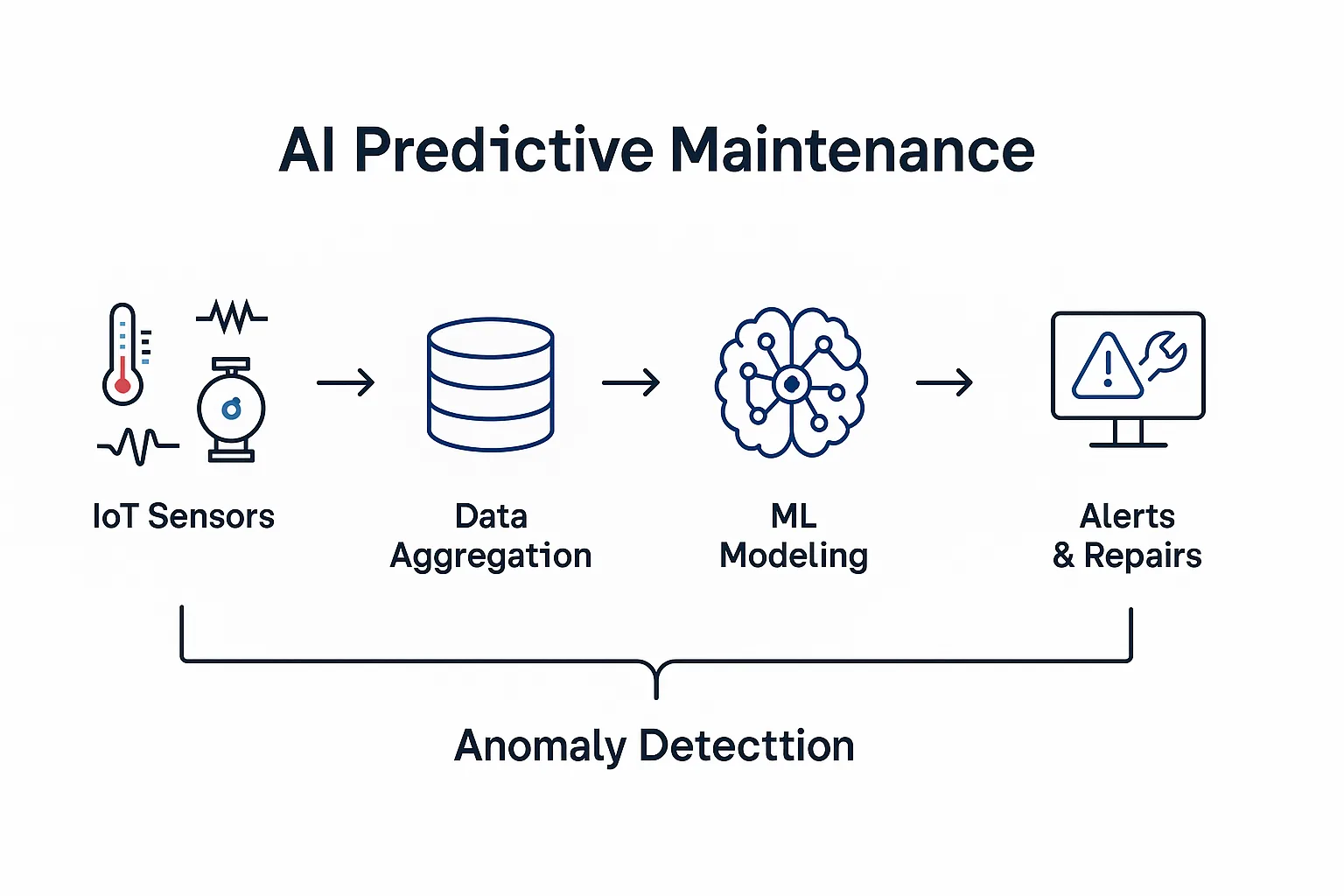
AI in predictive maintenance uses a blend of sensor-based maintenance systems, machine learning for equipment failure prediction, and real-time data analytics. Here’s how it works:
- Data Collection: Industrial IoT maintenance systems use sensors to track temperature, vibration, load, and pressure.
- Modeling & Forecasting: Machine learning models learn normal equipment behavior and flag anomalies.
- Prescriptive Action: The system suggests the best times for repairs or replacements.
This is AI-driven asset management in action—context-aware, timely, and predictive.
How Predictive Maintenance Directly Reduces Downtime
By continuously monitoring assets, AI enables real-time equipment monitoring that identifies small issues before they escalate. Benefits include:
- Early failure detection: Prevents costly breakdowns
- Optimized maintenance scheduling: Avoids peak production hours
- Extended asset lifespan: Through targeted interventions
- Reduced emergency repairs: Less chaos, more control
This proactive, AI for predictive maintenance in manufacturing approach keeps machines running longer and more reliably.
Financial Impact of Predictive Maintenance: ROI and Cost Savings Metrics
The return on investment from predictive maintenance is not theoretical—it’s proven:
- 50% reduction in unplanned downtime
- 30% decrease in overall maintenance costs
- 70% fewer equipment failures
- 20–40% extension of equipment lifespan
With CMMS integration with AI, manufacturers gain a centralized, intelligent platform for scheduling and tracking maintenance, driving stronger financial outcomes.
Predictive Maintenance Use Cases from the Field
Real-world applications demonstrate the versatility and value of predictive maintenance:
- Caterpillar: Uses AI to monitor equipment health, reducing field failures
- Rio Tinto: Applies predictive algorithms to manage asset wear
- BASF: Leverages machine learning for equipment failure prediction in chemical production
- DHL: Implements sensor-based maintenance systems to prevent conveyor belt delays
These predictive maintenance examples highlight how AI for predictive maintenance improves reliability across sectors.
From Pilot to Full Deployment: AI in Predictive Maintenance Implementation Roadmap
Here’s how to implement predictive maintenance:
- Evaluate current equipment and data infrastructure
- Install IoT sensors on key machinery
- Build real-time data pipelines to cloud or edge platforms
- Train models with both historical and live data
- Enable CMMS integration with AI for seamless maintenance scheduling
- Scale incrementally based on ROI and early wins
This phased approach builds confidence and ensures long-term success.
Predictive Maintenance Challenges and How to Overcome Them
While promising, predictive maintenance does pose challenges:
- Data quality and volume: Focus first on high-value assets
- System integration: Use open APIs and middleware
- Change resistance: Show clear ROI and operational benefits
- Skill gaps: Upskill staff or partner with AI specialists
Companies that address these proactively are more likely to succeed with AI in predictive maintenance.
Strategic Value for the C-Suite
Predictive maintenance delivers value at every leadership level:
- CFOs: Smoother budgets, reduced capital expenditures
- COOs: Greater operational reliability
- CTOs: Progress toward digital transformation
- CEOs: Stronger resilience and innovation positioning
This is not just maintenance—it’s a strategic investment in smart manufacturing. In a world of unstable supply chains and rising operational costs, reactive strategies no longer suffice. Early adopters of AI-based predictive maintenance aren’t just staying ahead—they’re redefining industry standards. With predictive analytics in manufacturing, companies are shifting from firefighting to forecasting.
Increase Uptime with Beamdata’s Supply Chain Management
Beam Data provides a turnkey solution to deploy AI for predictive maintenance quickly:
- Customized ML models tailored to your operations
- End-to-end sensor-based maintenance systems
- Dashboards and alerts for real-time equipment monitoring
- Seamless CMMS integration with AI for action and tracking
Our framework accelerates success from pilot to full deployment. Ready to transform your maintenance strategy? Partner with Beam Data to unlock the full power of AI and eliminate unplanned downtime before it impacts your bottom line. We’ve worked with manufacturers across industries to integrate AI-based predictive maintenance into their existing operations—quickly, effectively, and with measurable ROI.
Every manufacturing environment is unique—and so are its maintenance challenges. At Beam Data, we don’t believe in one-size-fits-all. Our team collaborates with you to design custom sensor-based maintenance systems, data pipelines, and machine learning models aligned to your specific equipment, workflows, and operational goals. Whether you’re dealing with legacy machines, diverse data sources, or scaling plant-wide solutions, we build systems that fit—never force.
AI in Predictive Maintenance FAQs
What types of data are needed to start predictive maintenance?
Common inputs include vibration data, temperature readings, usage cycles, and failure history via industrial IoT maintenance systems.
How soon can ROI be expected after deployment?
Typically within 6–12 months, depending on asset criticality and system readiness.
Can predictive maintenance be used for legacy equipment?
Yes—sensor-based maintenance systems and smart AI retrofits make it possible.
How does Beam Data integrate with our existing ERP or CMMS?
We provide seamless CMMS integration with AI via APIs and middleware.
Is AI predictive maintenance suitable for mid-sized manufacturers?
Absolutely—AI for predictive maintenance in manufacturing scales effectively for businesses of any size.